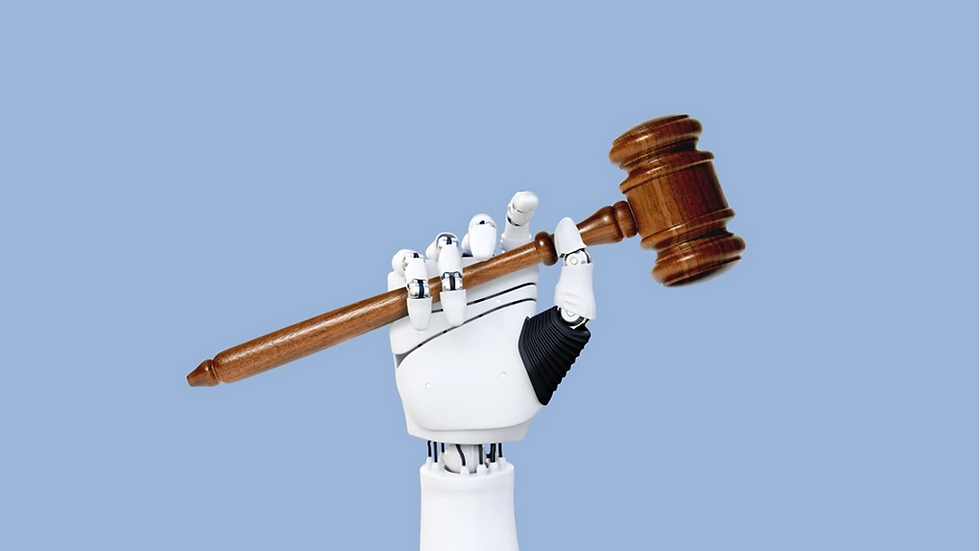
Introduction
An asylum tribunal hearing I attended last year in Glasgow, featuring an online witness, saw protracted technical difficulties. The judge jokingly pointed out how claims of artificial intelligence (AI) soon replacing asylum judges remained ludicrous as long as even court video conferencing continued to malfunction.
He may have been right–the asylum process is still far from being fully automated. Nonetheless, although still in their infancy, AI technologies are evolving exponentially (Bornet, 2023), along with their public and private sector appeal (Forster, 2022). The 2024 EU Artificial Intelligence Act (AI Act) lists eight high-risk areas for AI use (Artificial Intelligence Act, 2024, Annex III). It requires AI systems to undergo thorough inspections before being made available in the EU in these eight areas. One of them is “migration, asylum and border control management” (EU AI Act, 2023). The closest UK equivalent of this Act, a policy paper titled ‘A Pro-Innovation Approach to AI Regulation’, sets forth several pre-deployment non-binding principles for AI, including safety, transparency, fairness, accountability, and contestability, without referencing asylum (A Pro-Innovation Approach to AI Regulation, 2023, Section 3.2.3).
Despite this regulatory gloss, I believe the use of AI as humanitarian tech in asylum contexts comes with structural flaws that undermine claimants’ access to justice. Building on Gill et al.’s emphasis of non-material hurdles to fairness in asylum hearings (Gill et al., 2021, p. 62), I focus on the feasibility and unseen implications of AI on the fairness of asylum proceedings. I consider two areas where AI is discussed or already implemented, namely credibility assessments and court translations. I explore three issues: lack of trauma awareness, epistemic injustice, and errors in court translations. Given the ever-shifting nature of AI law, I nuance my arguments using examples from multiple jurisdictions, namely the UK, the EU, and the US.
Trauma and Inconsistencies
First, under the new standard of proof in UK asylum law‒the balance of probabilities‒set forth by the 2022 UK Nationalities and Borders Act, asylum claimants shoulder more of the burden of proof in credibility assessments today than they did before 2022. Minor discrepancies in claimants’ stories are now considered sufficient grounds to indicate false claims (False Representations: Caseworker Guidance, 2020, p. 14). This happens despite research proving that narrative inconsistencies often result from trauma (Herlihy et al., 2002, p. 325). Trauma impacts both the applicant’s ability to recollect events and their demeanour (VanPilsum-Bloom, 2022). A comprehensive online medical database review revealed that about 31.46% of refugees and asylum seekers suffer from post-traumatic stress disorder (PTSD) (Blackmore et al., 2020, p. 10). The disorder is, however, complicated to prove, involving significant financial and logistical resources for claimants. It not only requires a GP's medical decision but also evidence of a treatment plan developed with a mental health professional (Medical Claims under Articles 3 and 8 of the European Convention on Human Rights (ECHR), 2020, p. 33). Moreover, the current UK Government trauma-informed practice guidelines on dealing with migrants suffering from PTSD are only tailored for healthcare practitioners (Assessing New Patients from Overseas, 2021). The only mention of PTSD by the UK Home Office relates to how medical evidence should be produced to prove the condition (Medical Evidence in Asylum Claims, 2022). There is no official acknowledgement of how trauma can alter memory and spark inconsistencies in migrant testimonies. Nor are there guidelines on how asylum decision-makers should navigate such cases, except in claims of torture (ibid., p. 19).
Automating the asylum process would further aggravate this gap. Given the lack of a UK framework on how judges should consider the different recollection processes of trauma-affected asylum seekers when spotting discrepancies in their testimonies, this results in highly subjective assessments. Assigning this task to AI would require even more clear-cut instructions, as AI machinery builds on associations rather than a real understanding of concepts and situations (Bishop, 2021, p. 6). Rigorous mathematical instructions for asylum assessments are currently non-existent. Even if they existed, however, AI technologies would likely replicate the biases of their creators (Kinchin & Mougouei, 2022, p. 391). For instance, lie detectors are blind to trauma and bear Western-centric understandings of human behaviour when performing assessments (Westerling, 2022, p. 20). These risks far outweigh the potential advantages that AI could bring to asylum proceedings, such as pattern recognition, consistency, and predictability (Kinchin & Mougouei, 2022, pp. 388-90).
Human oversight, as the EU AI Act suggests, may be the solution (Artificial Intelligence Act, 2024, Art. 14). This would, however, create difficulties of its own. First, on top of being “one of the big regulatory questions of our time” (Laux, 2023), human oversight requires various layers of control to be effective (idem). In high-risk contexts like asylum, this would prove more resource-intensive and time-consuming than the non-automated process. Second, human oversight could create an illusion of legitimacy–humans would have to oversee high-risk and complex technical systems, making accidents almost unavoidable (Koulu, 2020, pp. 729-730). This is even more so the case if the algorithm used is opaque or “black box”. Such algorithms create predictive models (i.e., models that predict future behaviour based on patterns in existing data) according to variables they automatically generate, often in highly complex patterns (Rudin and Radin, 2019). This makes it impossible for humans to understand them, including their very designers (idem). Therefore, using the mere presence of human oversight rather than proof of its effectiveness as a justification for AI use in asylum screenings would grant AI artefacts legitimacy that is not empirically backed. Undetected AI errors could generate accidental refoulement, causing grave human rights concerns.
Increased Epistemic Vulnerability
Second, different societies and their institutions produce knowledge in distinct ways. The powerful often decide which knowledge production systems they legitimise, creating a hierarchy that sparks epistemic vulnerability in those whose knowledge systems are classified as ‘inferior’ (Westerling, 2022, pp. 12-13). Unlike in other forums, such as criminal law, in asylum contexts, the burden of proof falls on the claimants. The power relations in asylum interviews and appeals are thus by definition staked against them, leaving them at an epistemic disadvantage. Not only are they approached from the outset with disbelief as they attempt to prove their story (Jubany, 2011), but they also have to legitimise their sources of knowledge, such as oral evidence, in front of asylum decision-makers. This happens because the legal system of the country where a refugee applies for asylum will set its criteria for assessing their story and evidence and determining its ‘authenticity’ (Westerling, 2022, p. 13). Such assessments fall within broader categories known as hermeneutical resources, understood as “shared resources for social interpretation” (Fricker, 2007). Hermeneutical resources are distributed unevenly among social groups and individuals, according to their power. In simple words, “the powerful” find it easier to make sense of their experiences and make them understandable to others, while “the powerless find themselves having social experiences through a glass darkly, with at best ill-fitting meanings to draw on in the effort to render them intelligible” (idem). Miranda Fricker coined the notion of “hermeneutical injustice” as a type of epistemic injustice, wherein “someone has a significant area of their social experience obscured from understanding owing to prejudicial flaws in shared resources of social interpretation” (idem).
These concepts find useful illustration in asylum settings. Suppose an asylum decision-maker uses “structurally flawed” hermeneutical resources (for instance under the assumption that Western-produced knowledge is universal and superior). In that case, it hinders claimants from contributing their own knowledge to the shared epistemic resources of the court. The system thus undermines their epistemic agency (Ferreira, 2023, p. 307). Not only are asylum claimants in a vulnerable position for having to turn their subjective experience into an objectively verifiable narrative. But they must do so on an unequal footing as their knowledge production is deemed inferior to that of the court, including the production of truth–a result of neocolonial and systemic violence. Asylum claimants are perceived as “suspect subjects”, trapped in a paradox where they are both suspected of lying and deemed incapable of truth (Lorenzini & Tazzioli, 2018, pp. 72-73).
AI would exacerbate this imbalance. By portraying data as impartial and contextless, AI creates the illusion of objective truth. This fallacy results from data overconfidence, where technology does not eliminate pre-existing bias, but merely obscures it (Westerling, 2022, pp. 20-22). Quantitative studies back this assumption, highlighting how humans tend to feel false comfort–or, in other words, overconfidence–“in an incorrect decision by receiving AI advice” (Taudien et al., 2022). As a result, because of the epistemic hierarchy that disadvantages the claimant and algorithmic bias concealed by its apparent objectivity, a sharp distinction would emerge between the state’s ‘clean’ data and the applicant’s ‘raw’ data, which stems from knowledge production systems specific to the applicant’s country of origin (Westerling, 2022, p. 21). Because of this, AI would render the burden of proof on applicants in automated asylum decision-making systems unreasonable (idem).
In response, scholars have explored error mitigation methods, such as listing the degree of certainty AI had in the information it provided (Taudien et al., 2022). Nonetheless, this only partly reduced the risk of error, while not increasing the overall accuracy of the decision (Fügener et al., 2021, p. 1543). In this regard, Art. 9(3) of the EU AI Act only encourages risk management strategies for risks “which may be reasonably mitigated or eliminated through the development or design of the high-risk AI system” (Artificial Intelligence Act, 2024, Art. 9(3)). For the time being, it is highly questionable whether this principle of reasonableness can be upheld when using AI in high-risk decision-making.
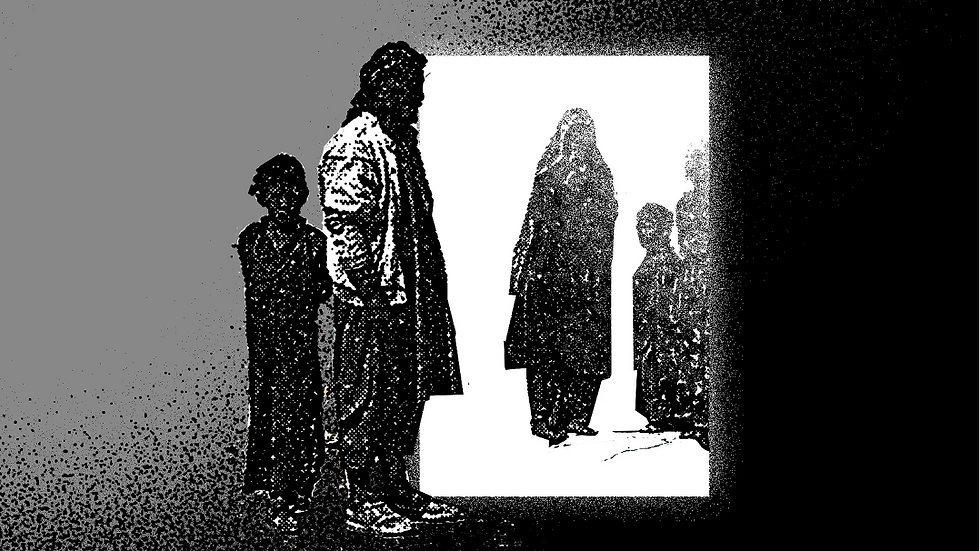
From Mistranslation to Deportation
The third reason why AI would hinder justice in asylum proceedings is translation errors. While an AI asylum judge may still sound outlandish, AI interpreters are on the rise. From government contracts with automated translation providers to the US Immigration and Customs Enforcement’s (ICE) use of Google Translate for refugees, machine translation is becoming common in the US asylum system (Bhuiyan, 2023). These tools are, however, not without flaws. For instance, AI translators tend to produce inaccurate translations due to insufficient data on rare languages and misunderstandings of cultural sensitivities (Stuber, 2020). In a country where the government will “weaponise small language technicalities to justify deporting someone”, the stakes are very high (Bhuiyan, 2023). And while the risk of error cannot be eliminated, AI will be more prone to mistakes than a qualified interpreter. This is because machine translation has no cultural awareness and cannot read between the lines in situations where literal translations make little sense (Yang et al., 2023, p. 6). Human translators, on the other hand, are more aware of context, cultural subtleties, and highly intricate cross-linguistic meaning discrepancies, including untranslatable metaphors or idioms (Deck, 2023).
Conclusion
To conclude, in this short article I warn against AI use in asylum decisions. I issue an indirect call to the EU and relevant international regulatory agencies to qualify asylum as an ‘unacceptable risk’ category for automation. While this may increase waiting times for claimants, it will ensure a minimal level of fairness in asylum decision-making. The words of the judge in the hearing I attended, addressing the asylum claimant, resonated with me: “Elon Musk might make it possible in the future to judge cases by AI, but today I will be judging your case on a personal level”.
References
A pro-innovation approach to AI regulation. (2023). [Policy Paper]. UK Government Department for Science, Innovation & Technology; Office for Artificial Intelligence. https://www.gov.uk/government/publications/ai-regulation-a-pro-innovation-approach/white-paper
Artificial Intelligence Act (2024). http://data.europa.eu/eli/reg/2024/1689/oj/eng
Assessing new patients from overseas: Migrant health guide. (2021, August 2). GOV.UK. https://www.gov.uk/guidance/assessing-new-patients-from-overseas-migrant-health-guide
Bhuiyan, J. (2023, September 7). Lost in AI translation: Growing reliance on language apps jeopardizes some asylum applications. The Guardian. https://www.theguardian.com/us-news/2023/sep/07/asylum-seekers-ai-translation-apps
Bishop, J. M. (2021). Artificial Intelligence Is Stupid and Causal Reasoning Will Not Fix It. Frontiers in Psychology, 11. https://doi.org/10.3389/fpsyg.2020.513474
Blackmore, R., Boyle, J. A., Fazel, M., Ranasinha, S., Gray, K. M., Fitzgerald, G., Misso, M., & Gibson-Helm, M. (2020). The prevalence of mental illness in refugees and asylum seekers: A systematic review and meta-analysis. PLOS Medicine, 17(9). https://doi.org/10.1371/journal.pmed.1003337
Bornet, P. (2023). How The Tech Sector Can Help Bridge The Divide Between Exponential Progress And Linear Thinking. Forbes. https://www.forbes.com/councils/forbestechcouncil/2023/04/13/how-the-tech-sector-can-help-bridge-the-divide-between-exponential-progress-and-linear-thinking/
Deck, A. (2023). AI translation is jeopardizing Afghan asylum claims. Rest of World. https://restofworld.org/2023/ai-translation-errors-afghan-refugees-asylum/
EU AI Act: First regulation on artificial intelligence. (2023, August 6). Topics | European Parliament. https://www.europarl.europa.eu/topics/en/article/20230601STO93804/eu-ai-act-first-regulation-on-artificial-intelligence
False representations: Caseworker guidance. (2020, January). GOV.UK. https://assets.publishing.service.gov.uk/media/65707268746930000d488908/Suitability+false+representations++.pdf
Ferreira, N. (2023). Utterly Unbelievable: The Discourse of ‘Fake’ SOGI Asylum Claims as a Form of Epistemic Injustice. International Journal of Refugee Law, 34(3–4), 303–326. https://doi.org/10.1093/ijrl/eeac041
Forster, M. (2022). Refugee protection in the artificial intelligence era: A test case for rights [Research Paper]. Royal Institute of International Affairs. https://doi.org/10.55317/9781784135324
Fricker, M. (2007) ‘Hermeneutical Injustice’, in M. Fricker (ed.) Epistemic Injustice: Power and the Ethics of Knowing. Oxford University Press. Available at: https://doi.org/10.1093/acprof:oso/9780198237907.003.0008
Fügener, A., Grahl, J., Gupta, A., & Ketter, W. (2021). Will Humans-in-the-Loop Become Borgs? Merits and Pitfalls of Working with AI (Open Access). MIS Quarterly, 45(3b), 1527–1556. https://doi.org/10.25300/MISQ/2021/16553
Gill, N., Allsopp, J., Burridge, A., Fisher, D., Griffiths, M., Paszkiewicz, N., & Rotter, R. (2021). The tribunal atmosphere: On qualitative barriers to access to justice. Geoforum, 119, 61–71. https://doi.org/10.1016/j.geoforum.2020.11.002
Herlihy, J., Scragg, P., & Turner, S. (2002). Discrepancies in autobiographical memories—Implications for the assessment of asylum seekers: Repeated interviews study. BMJ, 324(7333), 324–327. https://doi.org/10.1136/bmj.324.7333.324
Jubany, O. (2011). Constructing truths in a culture of disbelief: Understanding asylum screening from within. International Sociology, 26(1), 74–94. https://doi.org/10.1177/0268580910380978
Kinchin, N., & Mougouei, D. (2022). What Can Artificial Intelligence Do for Refugee Status Determination? A Proposal for Removing Subjective Fear. International Journal of Refugee Law, 34(3–4), 373–397. https://doi.org/10.1093/ijrl/eeac040
Koulu, R. (2020). Proceduralizing control and discretion: Human oversight in artificial intelligence policy. Maastricht Journal of European and Comparative Law, 27(6), 720–735. https://doi.org/10.1177/1023263X20978649
Laux, J. (2023). Institutionalised distrust and human oversight of artificial intelligence: Towards a democratic design of AI governance under the European Union AI Act. AI & Society. https://doi.org/10.1007/s00146-023-01777-z
Lorenzini, D., & Tazzioli, M. (2018). Confessional Subjects and Conducts of Non-Truth: Foucault, Fanon, and the Making of the Subject. Theory, Culture & Society, 35(1), 71–90. https://doi.org/10.1177/0263276416678291
Pinto, F. (2023) ‘Can AI Improve the Justice System?’, The Atlantic, 13 February. Available at: https://www.theatlantic.com/ideas/archive/2023/02/ai-in-criminal-justice-system-courtroom-asylum/673002/ (Accessed: 3 November 2024).
Rudin, C. and Radin, J. (2019) ‘Why Are We Using Black Box Models in AI When We Don’t Need To? A Lesson From an Explainable AI Competition’, Harvard Data Science Review, 1(2). Available at: https://doi.org/10.1162/99608f92.5a8a3a3d
Medical claims under Articles 3 and 8 of the European Convention on Human Rights (ECHR). (2020, 190). UK Home Office. https://assets.publishing.service.gov.uk/media/5f8dae7fd3bf7f49adb156b4/medical-claims-_article3and8_-v8.0ext.pdf
Medical evidence in asylum claims: Caseworker guidance. (2022, August 30). UK Home Office. https://assets.publishing.service.gov.uk/media/630ddf09e90e0729df07ad95/Medical_evidence_in_asylum_claims.pdf
Stuber, S. (2020, October 27). Interpretation gaps plague French asylum process. The New Humanitarian. https://www.thenewhumanitarian.org/news-feature/2020/10/27/france-migration-asylum-translation
Taudien, A., Fügener, A., Gupta, A., & Ketter, W. (2022). The Effect of AI Advice on Human Confidence in Decision-Making. Proceedings of the 55th Hawaii International Conference on System Sciences (HICSS).
VanPilsum-Bloom, L. (2022, April 18). An Illogical and Harmful Assessment: Credibility Findings in Trauma Survivor Asylum Applicants. Minnesota Journal of Law & Inequality. https://lawandinequality.org/2022/04/18/an-illogical-and-harmful-assessment-credibility-findings-in-trauma-survivor-asylum-applicants/
Westerling, F. A. (2022). Technology-related Risks to the Right to Asylum: Epistemic Vulnerability Production in Automated Credibility Assessment. European Journal of Law and Technology, 13(3). https://ejlt.org/index.php/ejlt/article/view/891
Yang, Y., Liu, R., Qian, X., & Ni, J. (2023). Performance and perception: Machine translation post-editing in Chinese-English news translation by novice translators. Humanities and Social Sciences Communications, 10(1), 798. https://doi.org/10.1057/s41599-023-02285-7
Comments